Exploring the Impact of AI Tools on Equity: A Reflection Using Chat GPT-3.5 as a Reference
By Ezra Tefera MD, MSc
Ezra Tefera is our first Heller community contributor. We encourage all Heller students, alumni, staff, and faculty to write to us at openair@brandeis.edu. We are committed to publishing letters that contribute to constructive dialogue among the Heller community.
These articles are solely a reflection of the contributor and are not endorsed by the Open-Air Journal or the Heller School.
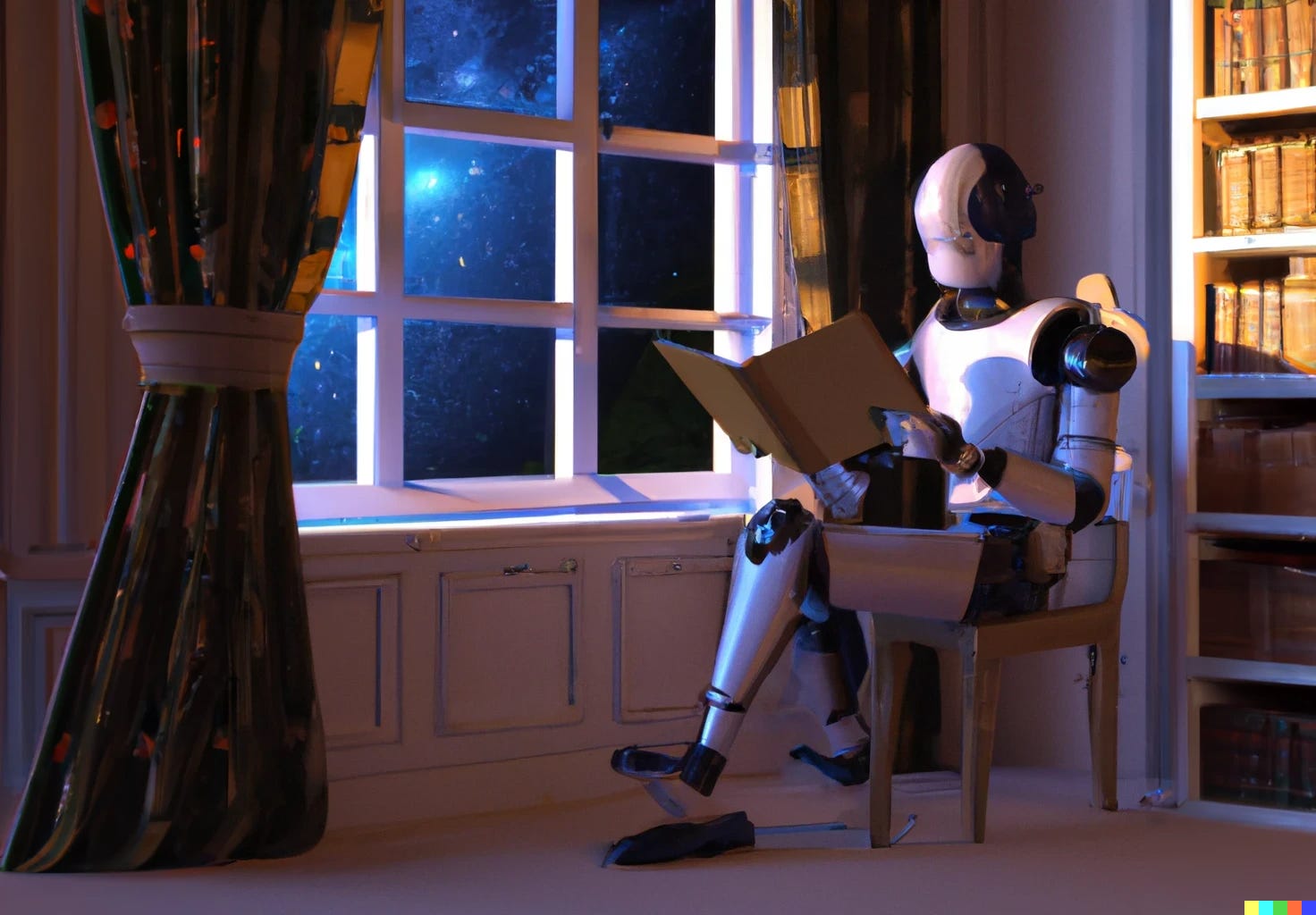
For a greater comprehension of AI and its language, jump to the end for a review of some of the fundamental terms.Â
The ongoing development of Artificial Intelligence (AI) tools has spurred a great conversation around their potential perks and drawbacks. This blog will explore AI applications such as Chat GPT 3.5, discussing the advantages and challenges of such technology.Â
Most recently, the release of Chat GPT-3.5 has sparked a lot of excitement and interest in the AI community, as well as society at large, because of its potential to change the way we interact with technology and revolutionize many industries. In January 2023, it was estimated to have 100 million monthly active users making it the fastest growing consumer app in history. Chat GPT 3.5 is now one of the most popular Natural Language Processing (NLP) models. In fact, Microsoft invested $10 billion s on OpenAI recognizing its business and application potential. It uses 300 billion words collected from the internet, books, articles, websites, and posts and provides services such as summarizing, writing conclusions, citation, paraphrasing, translating language, writing policy memos, outlining, and comparing papers, correcting writing style and grammar errors. Users also have the option to interact with upvoting and downvoting options.
Despite being a powerful tool, Chat GPT 3.5 and other similar AI tools have many limitations and concerns, such as misinformation, inaccurate or biased outputs, and harmful instructions. Additionally, such chatbots have an upvoting and downvoting reward model, which is meant to enhance the accuracy and pertinence of its responses. Nonetheless, this reward model system relies on human feedback which can inject human bias into the equation. For instance, an individual may be more likely to vote up or down based on their own personal perspective and prejudice rather than the exactness or appropriateness of the answer. This can result in an uneven and biased reward system, which may influence the chatbot's answers. Furthermore, propriety and copyright concerns, collecting sensitive information without consent fueling privacy issues, commercial interests, and improper compensation procedures are serious concerns of Chat GPT and similar AI tools. Most importantly, access limitations to underserved communities due to paywalls and other barriers is exacerbating inequitable and unfair use of technologies, to a greater extent amplifying systemic disparities.Â
The rapid growth of AI technology has had a significant effect on these underserved populations, and the consequences of this have been made clear through the systemic biases that manifest in technology transitions. These transitions often leave behind marginalized communities. This is noted in the recent AI tools as a result of the embedded biases found in their algorithms. This bias can be present at any stage of development, from data selection to the deployment of algorithms in contexts they weren't intended for, and the extent of the consequences depend on the type of data used in training. Below are the five major categories of algorithmic biases:
Dataset bias: Diversity of the client base is not well represented in data training machine learning algorithms.
Association bias: Cultural bias is reinforced and amplified by data used to train a model.
Interaction bias: AI is tampered with by humans, leading to skewed results.
Automation bias: Social and cultural elements are ignored by computerized decisions.
Confirmation bias: Overly simplistic personalization makes prejudicial judgments about a person or a group.
Furthermore, there are still many unanswered questions such as who is determining if the AI outputs are suitable, correct, and free from harm? What mechanisms are in place to check AI outputs, and can the information generated by AI be used and deployed? How does AI generate its answers when the 'black box effect' is still not fully understood? How current is the data used to train these algorithms?
The bias is especially evident in sensitive fields such as the healthcare sector, as marginalized communities are underrepresented in the Electronic Health Records (EHRs) used to train AI tools. This means that the models will not perform as well in underrepresented groups as they will in represented ones. This bias is also seen in self-driving cars, where pedestrian tracking was found to be less accurate in identifying individuals with darker skin tones, resulting in a much higher risk for those pedestrians. Additionally, hiring algorithms have been found to default towards bias of certain genders and races, amplifying existing prejudices.
There is a great deal of controversy over the techniques used to reduce algorithmic biases. One of these techniques, "reinforcement learning with human feedback", is used for "aligning" AI with human values and is found in tools such as Chat GPT. However, an article released by Time in January 2023 exposed how this method relies on the exploitation of labor in developing countries, wherein individuals are paid approximately $2/hour to be exposed to hazardous material in order to teach the AI how to avoid it. Additionally, several recent studies have suggested that reinforcement learning methods used to cleanse AI of racism, in fact, end up diminishing or eliminating the voices of marginalized communities.
In order to ensure algorithmic justice, a comprehensive regulatory mechanism should be implemented to both protect against the potential harms of AI tools and maximize their benefits in an equitable fashion. This would entail making licensing and continual monitoring of AI tools mandatory, instituting accountability and liability through a system such as the tort system, generating evidence for policy decisions through research, advocating for safer use of AI tools, and providing additional precautions in vulnerable sectors such as healthcare. This might involve supervising healthcare facilities to make sure that concerns like bias in training, security, and data privacy are addressed, training healthcare professionals, and developing/adopting and enforcing institutional guidelines/rules/policies. Moreover, it is important to understand and interpret the unknown factors that make up the "black box" of AI systems in order to better regulate them, and this could be achieved through institutionalized regulations rather than allowing AI companies to self-regulate.
Definitions
Artificial Intelligence (AI): AI is a broader category of technologies that allows machines to imitate the way humans think, learn, and act. It is an umbrella term that encompasses Natural Language Processing (NLP), Deep Learning (DL), and Machine Learning (ML)
Machine Learning (ML): AI and Machine Learning (ML) are sometimes used interchangeably, although this is a debated topic since AI existed before ML. However, Machine Learning is a subset of AI that uses algorithms to aid computers to learn from training data by identifying patterns and making predictions. Whereas Deep Learning is a subset of Machine Learning which uses multi-layered artificial neural networks to emulate the functioning of the human brain. The DL algorithms can recognize speech, analyze images, and understand natural language tex
Natural Language Processing (NLP): NLP is a division of AI that deals with the connection between computer systems and people with natural language. NLP involves devising algorithms and models that allow computers to process, comprehend, and generate language like humans. NLP is employed for activities such as text summarization or explanation, context evaluation, machine translation, identification, and responding to queries based on user requests. Lastly, Large Language Models (LLMs) are a kind of AI model that has been educated on a huge set of text data to create and anticipate human language.Â
Narrow vs General AI: AI models can also be further categorized based on their functionality type. Narrow AI systems perform only one, narrowly defined task or a set of related tasks such as Chat GPT 3.5 while General AI systems can carry out multiple intelligent tasks demonstrating intelligent behavior across a range of topics.